Background
Sergei Mikhailovich Prokudin-Gorskii (1863-1944) [Сергей Михайлович Прокудин-Горский, to his Russian friends] was a man well ahead of his time. Convinced, as early as 1907, that color photography was the wave of the future, he won Tzar's special permission to travel across the vast Russian Empire and take color photographs of everything he saw including the only color portrait of Leo Tolstoy. And he really photographed everything: people, buildings, landscapes, railroads, bridges… thousands of color pictures! His idea was simple: record three exposures of every scene onto a glass plate using a red, a green and a blue filter. Never mind that there was no way to print color photographs until much later. He envisioned special projectors to be installed in “multimedia” classrooms all across Russia where the children would be able to learn about their vast country. Alas, his plans never materialized: he left Russia in 1918, right after the revolution, never to return again. Luckily, his RGB glass plate negatives, capturing the last years of the Russian Empire, survived and were purchased in 1948 by the Library of Congress. The LoC has recently digitized the negatives and made them available on-line.
The goal of this project is to take the digitized Prokudin-Gorskii glass plate images and, using image processing techniques, automatically produce a color image with as few visual artifacts as possible. In order to do this, I extract the three color channel images, place them on top of each other, and align them so that they form a single RGB color image.
First Trial
We first tried to align the RGB channels with normalized intensity of each channel using the NCC metric. We first implemented the full-resolution brute-force alignment pipeline that traverses all the posible 2D per-pixel translations. Then we implemented a image pyramid alignment pipeline, that first align the coarse version of the image, then use the coarse offset as an anchor to search for the fine offset. The image pyramid alignment method significantly increases the alignment performance.


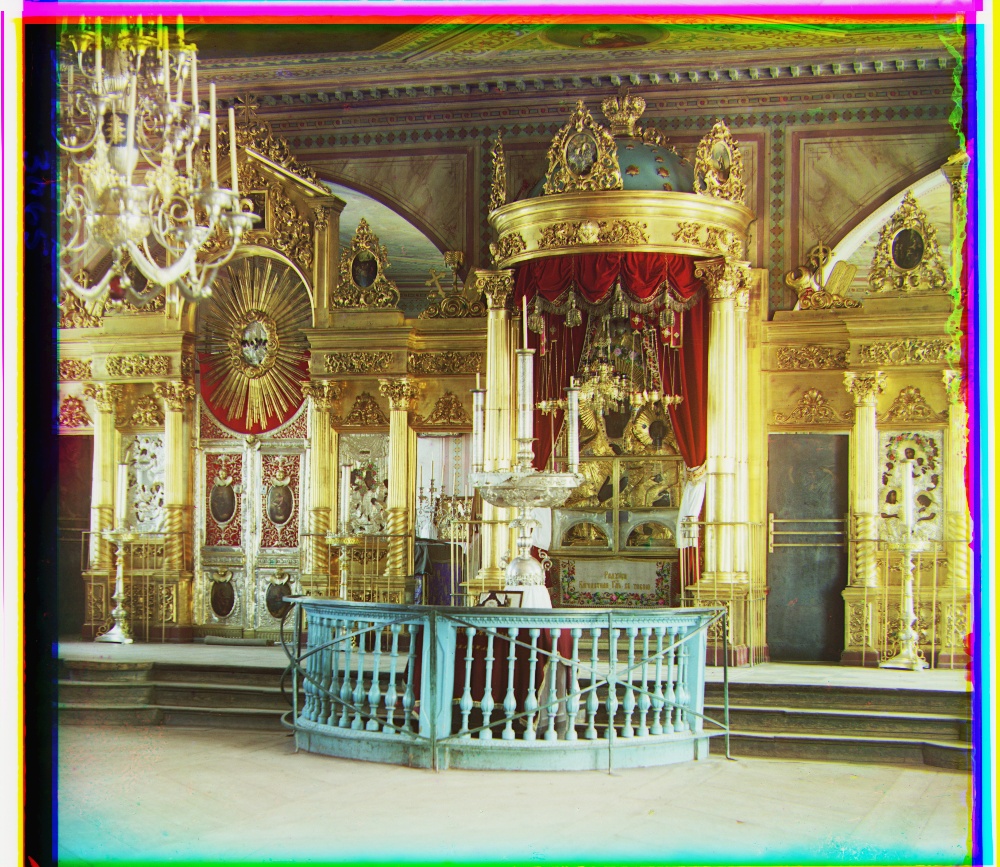
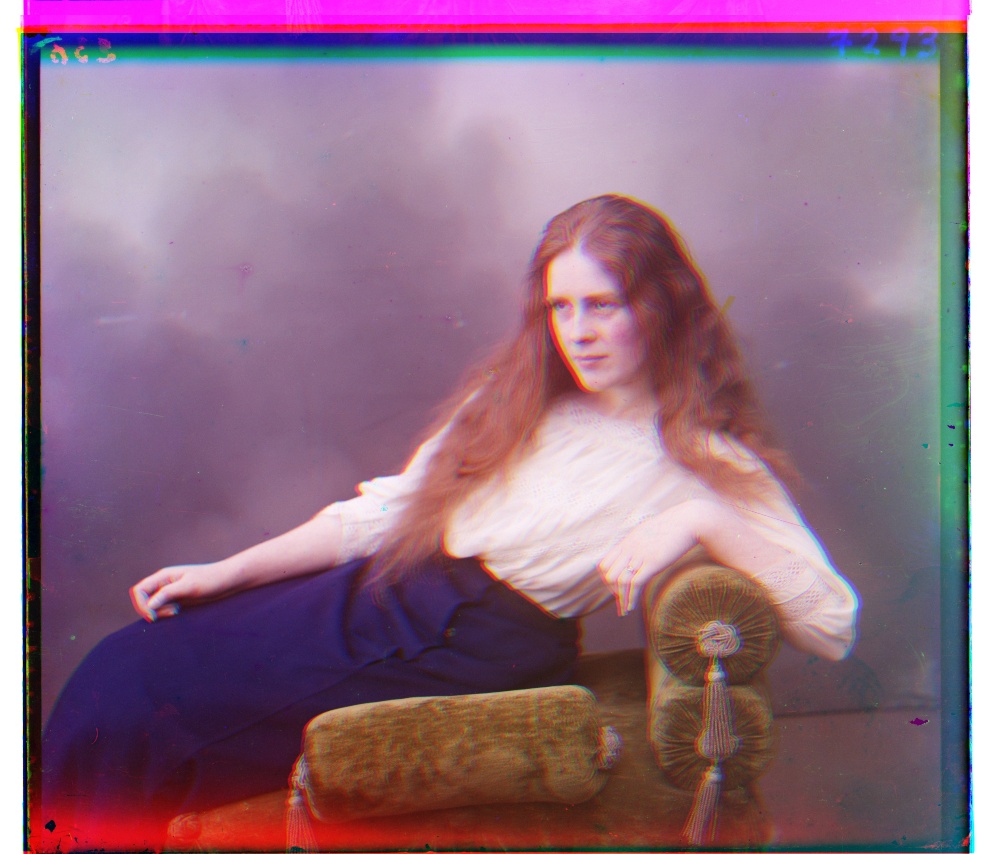


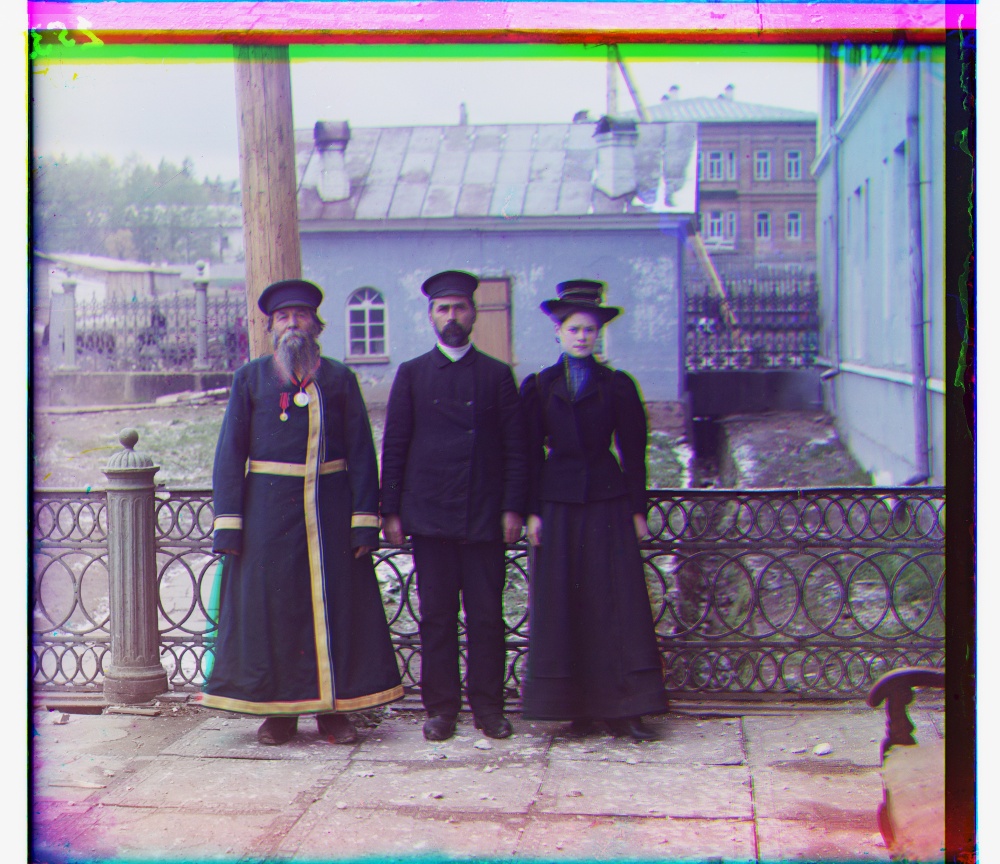
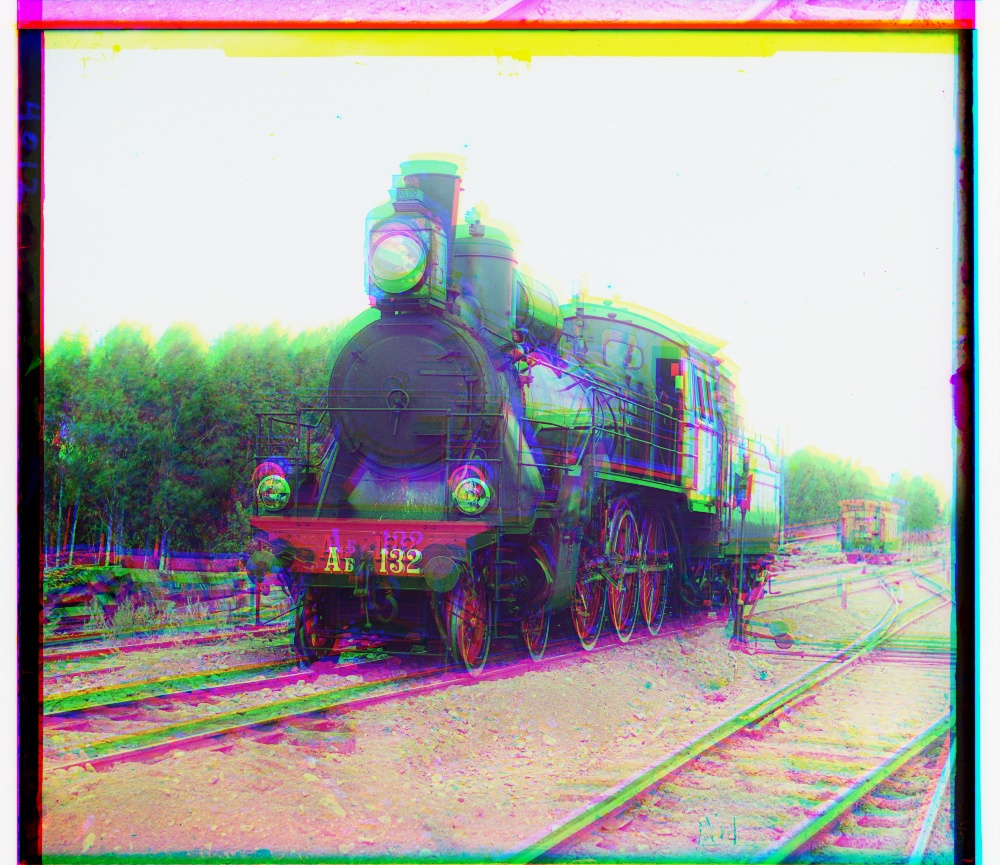
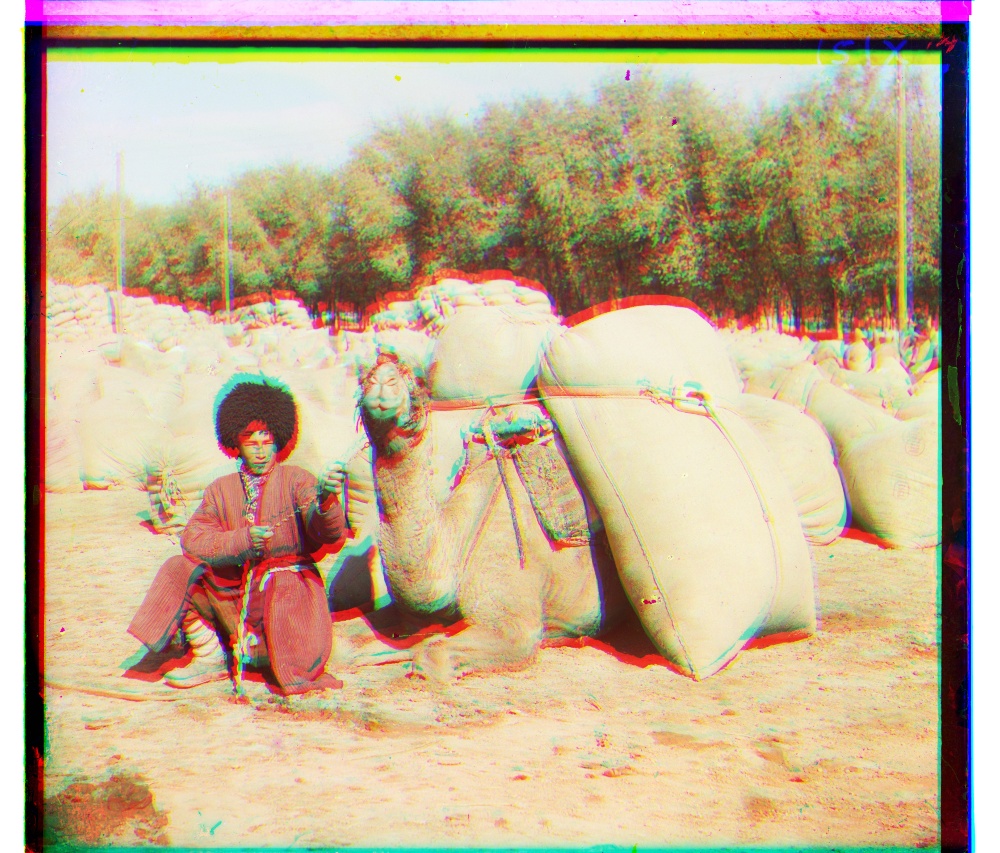
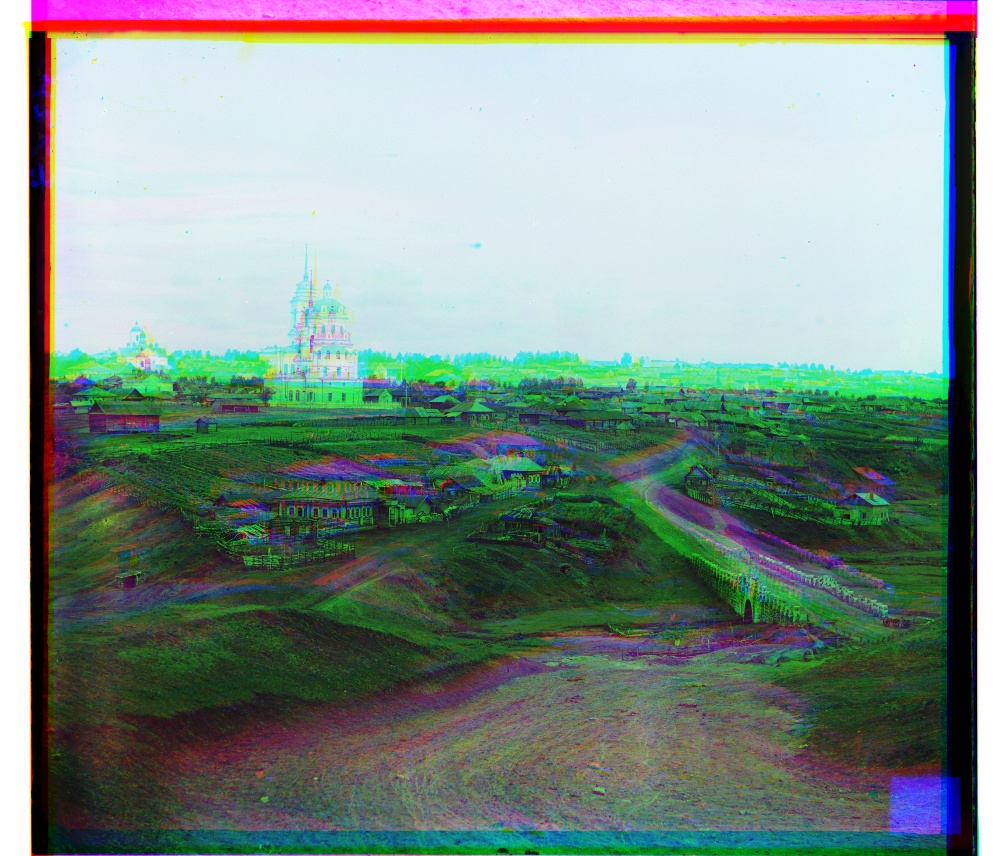
We observed that the alignment of some images is really not ideal. This is because the RGB intensity may not be consistent among those red, green and blue image channels. For example, in the Emir image, there are blue and red patterns on Emir's clothes. In those blue regions, the blue channel has very high intensity, while the red and green channels may have lower intensity in those blue areas.
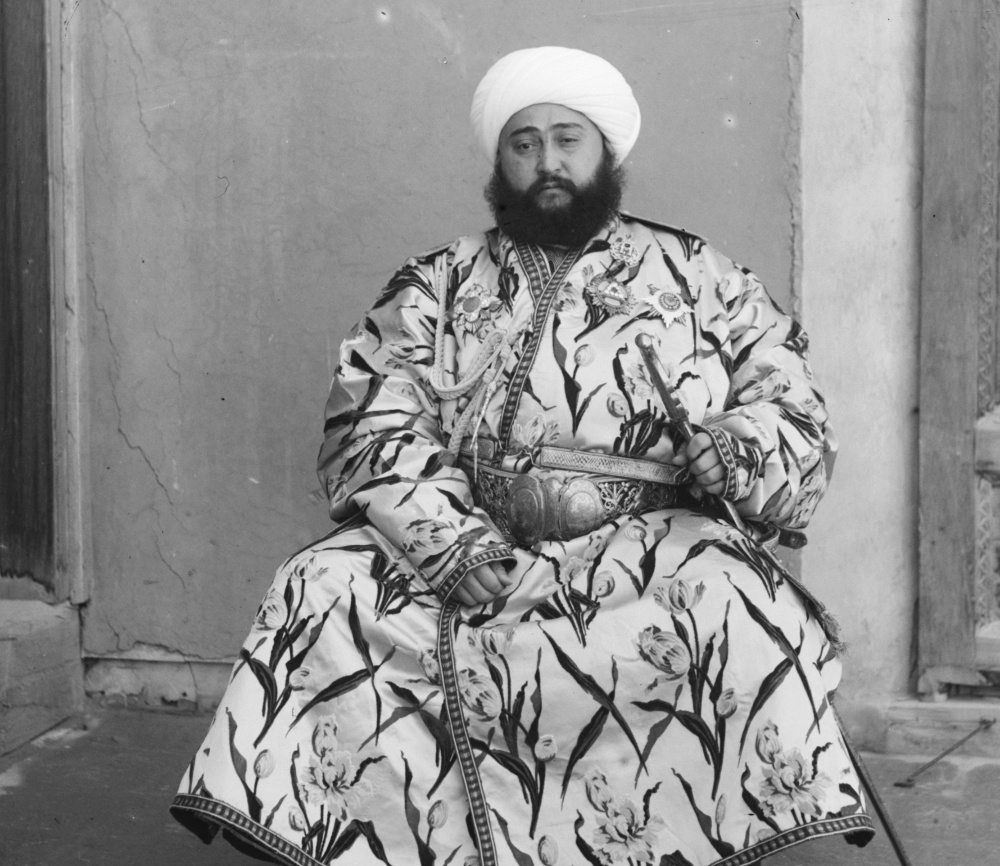
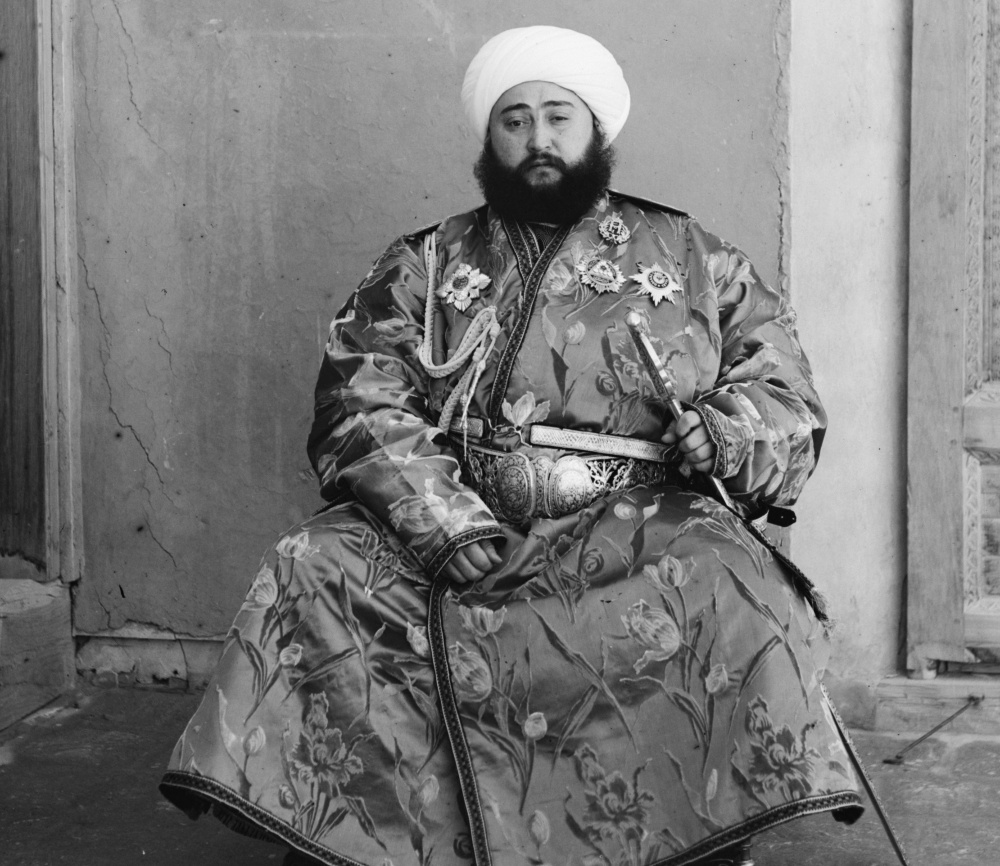

Align with Edges!
Here I implemented an edge detector to detect edges on all three R, G, B channels, and do the alignment depending on edge maps. Even though the intensity may be inconsistent as stated above, the edge maps across different channels are more consistent. For the implementation of the edge detector, we computed the gradient map gx, gy on x, y direction respectively, then compute the gradient intensity $g=\sqrt{g_x^2+g_y^2}$ and direction $\theta=\tan^{-1}(\frac{g_y}{g_x})$, then we do non-maximum supression along the gradient direction to make sure the edges that we detected are thin and precise.
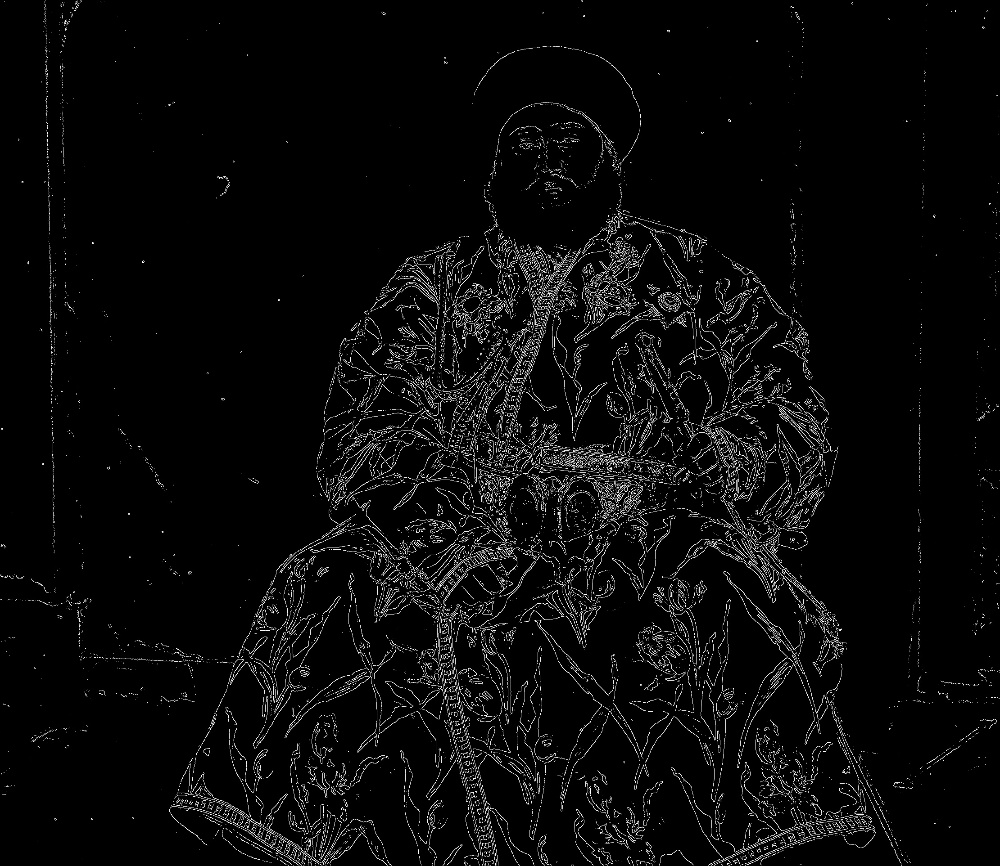


The alignment qualty has been improved a lot when we do alignment depending on the edge maps from different color channels. Here are some comparison results to show the quality improvement from edge alignment.

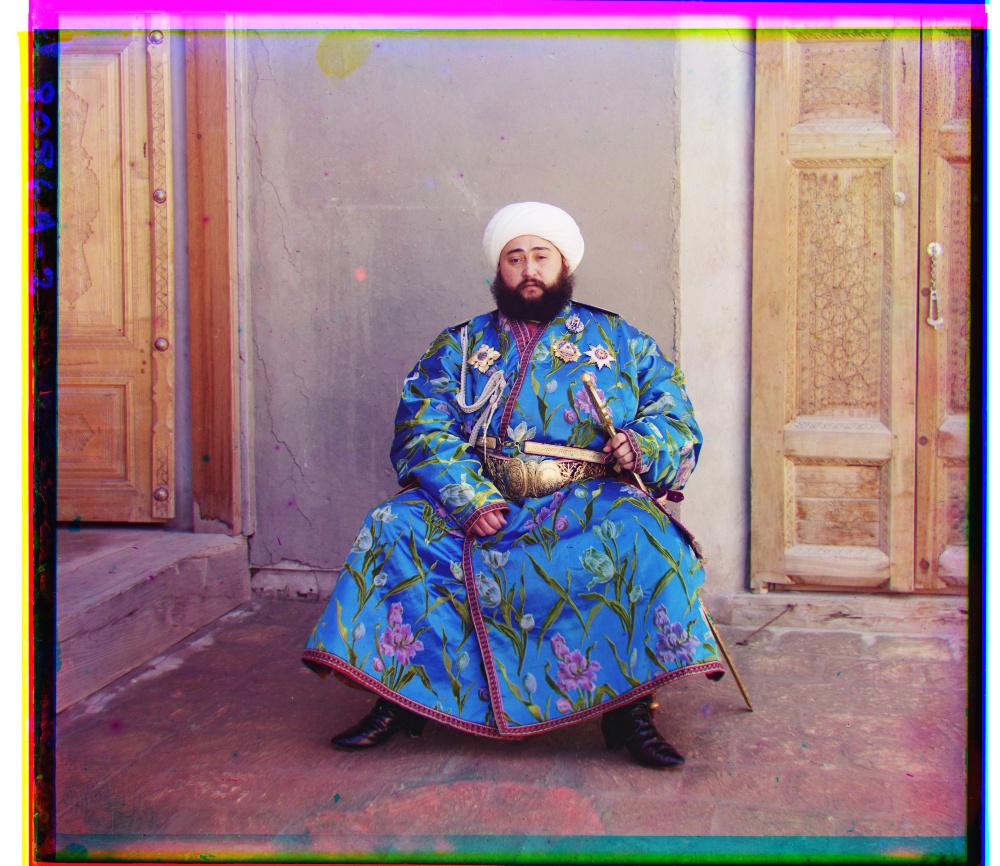

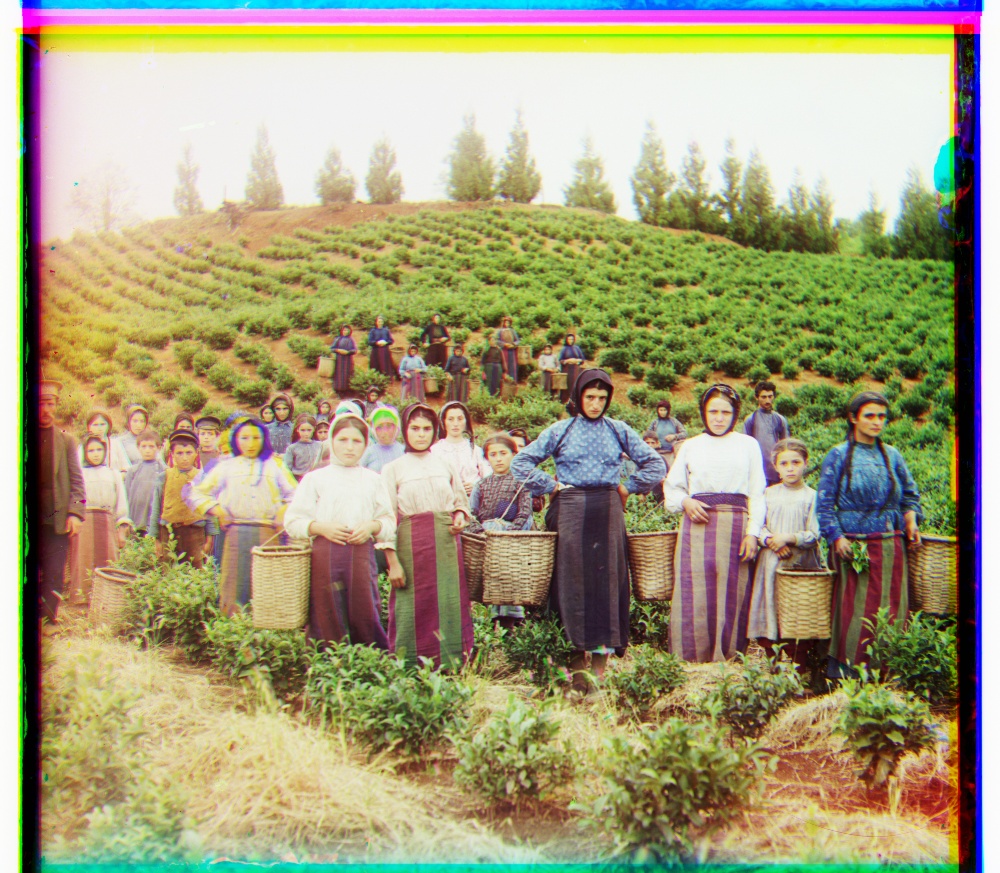

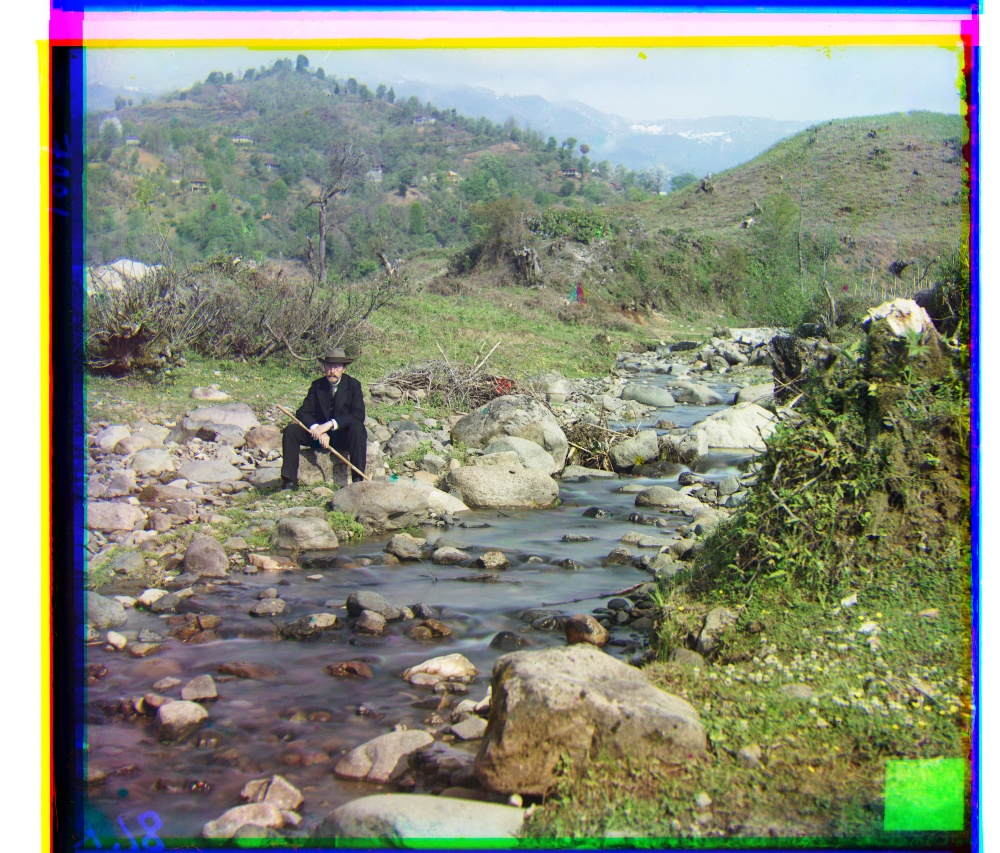
Auto Contrasting
I find that the result RGB images looks pale. So I use sine function to increase the contrast of the images automatically.
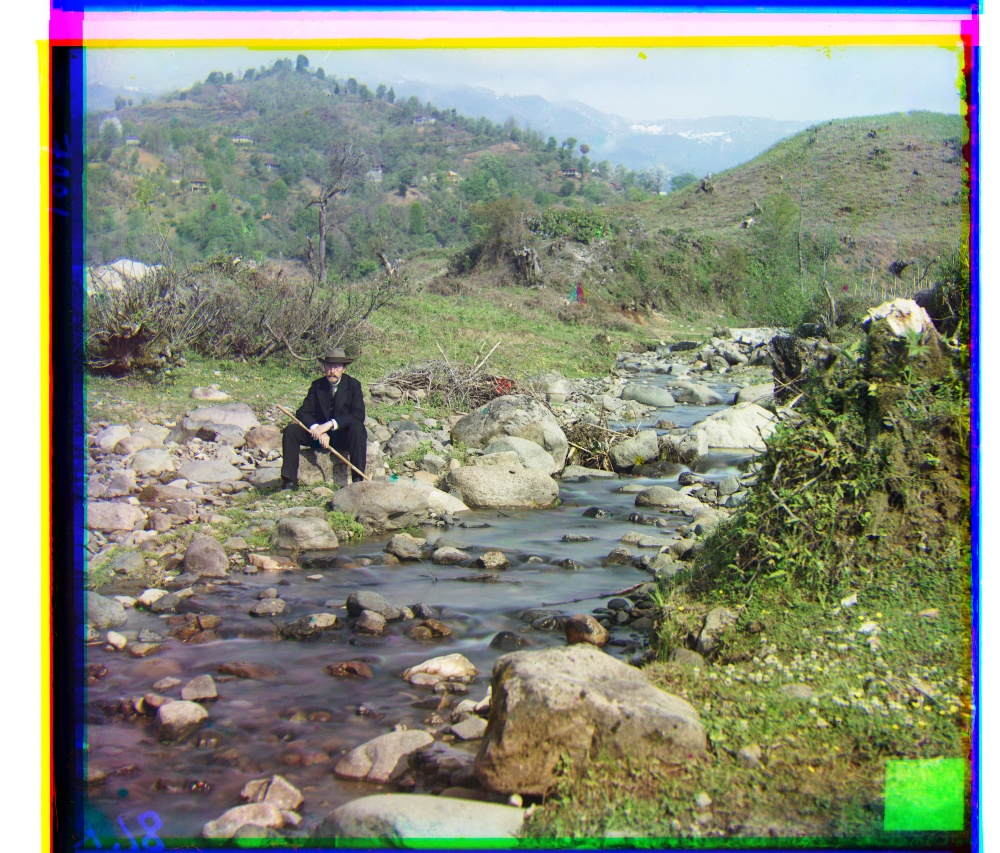

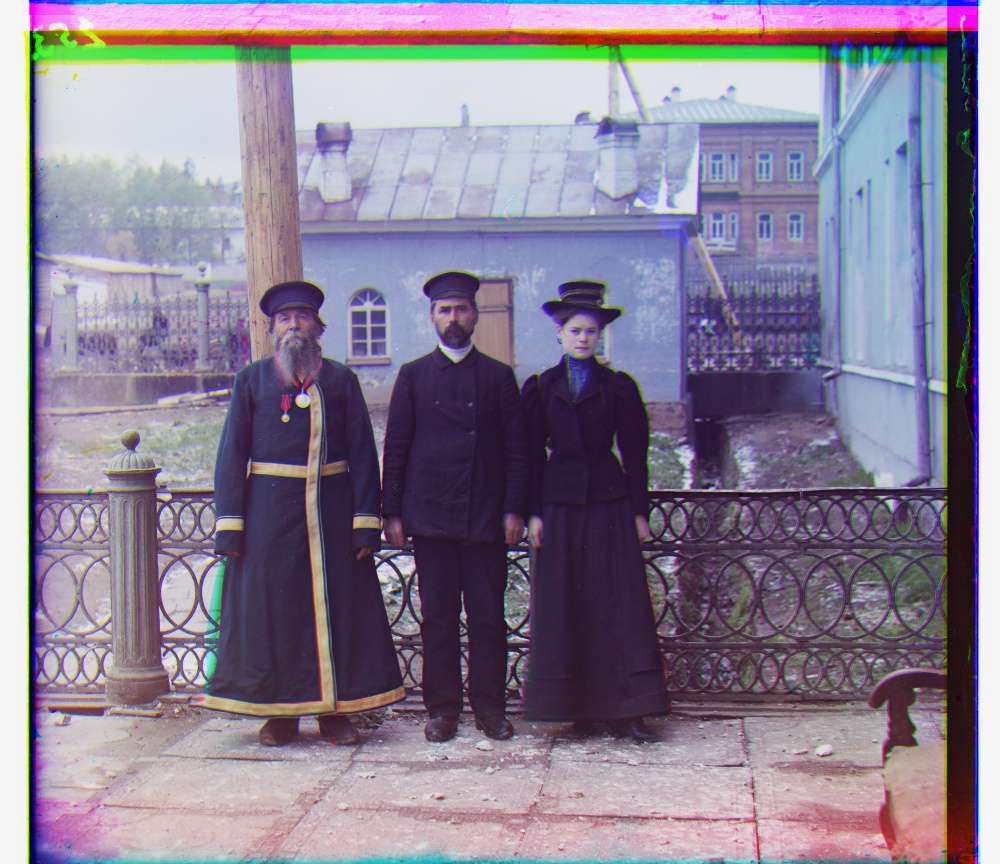
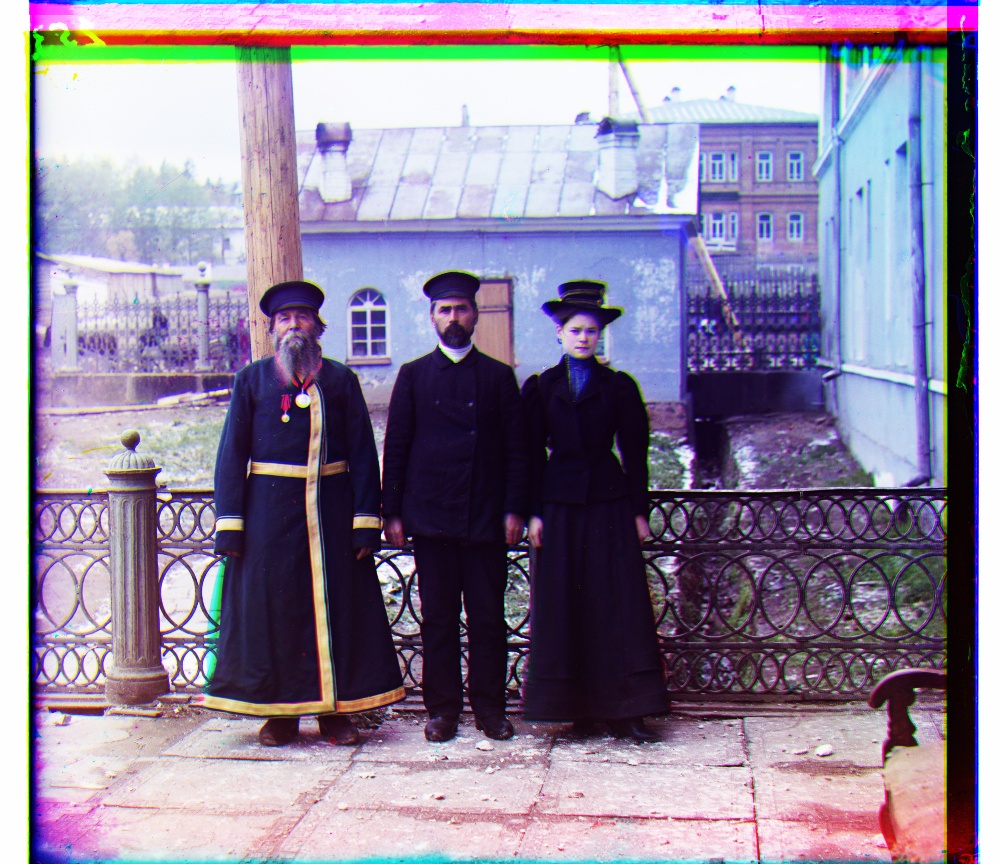
Auto Color Mapping
I find that simply overlapping the original R,G,B channels produces unnatural color results. So, I implemented a automatic color mapping to balance the energy of R, G, B channels in order to produce more natural color, especially the skin color.


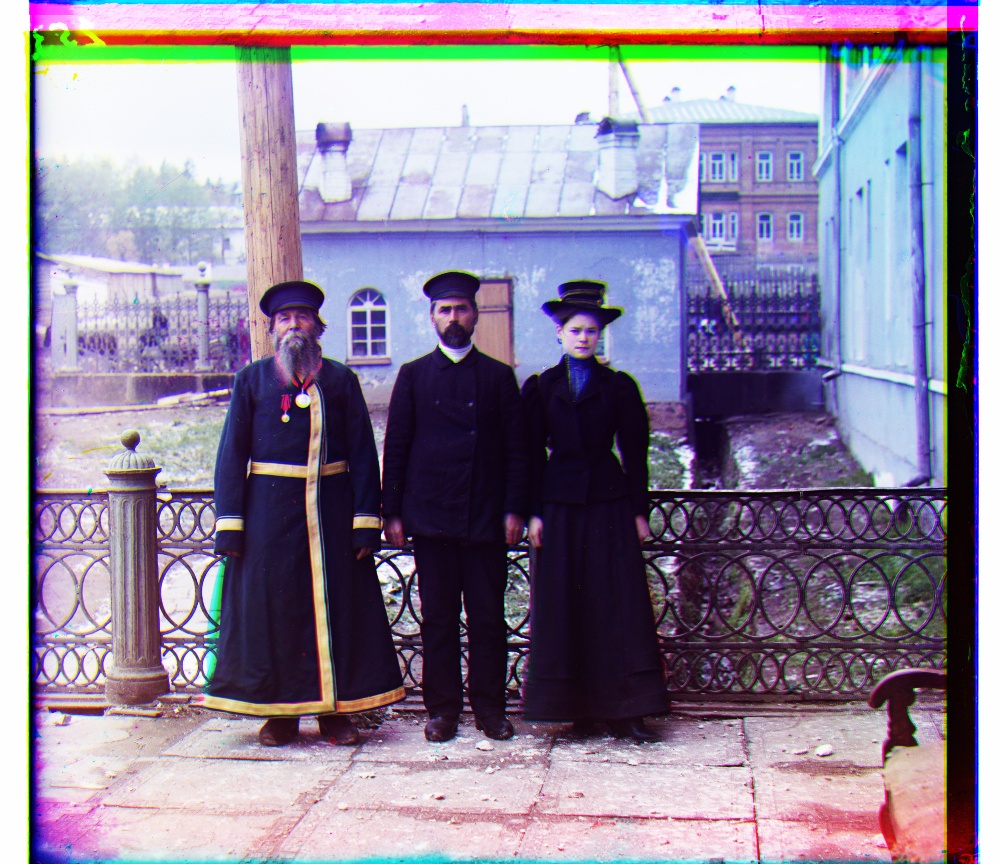

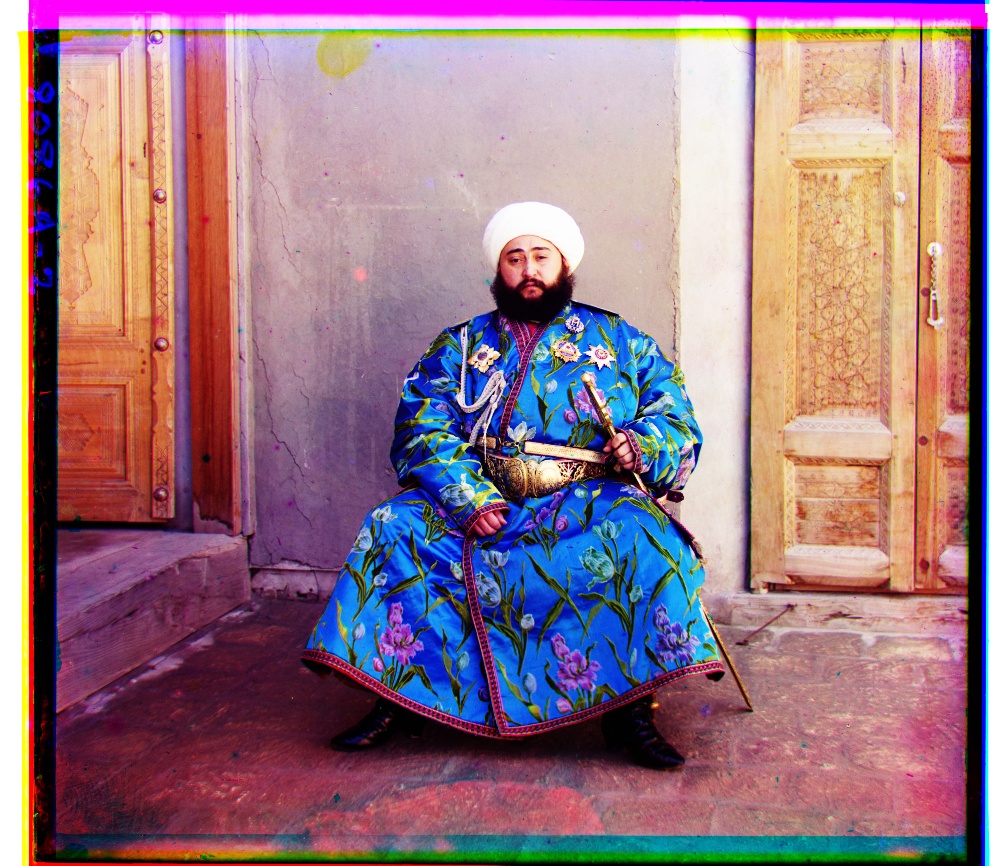

Gallery

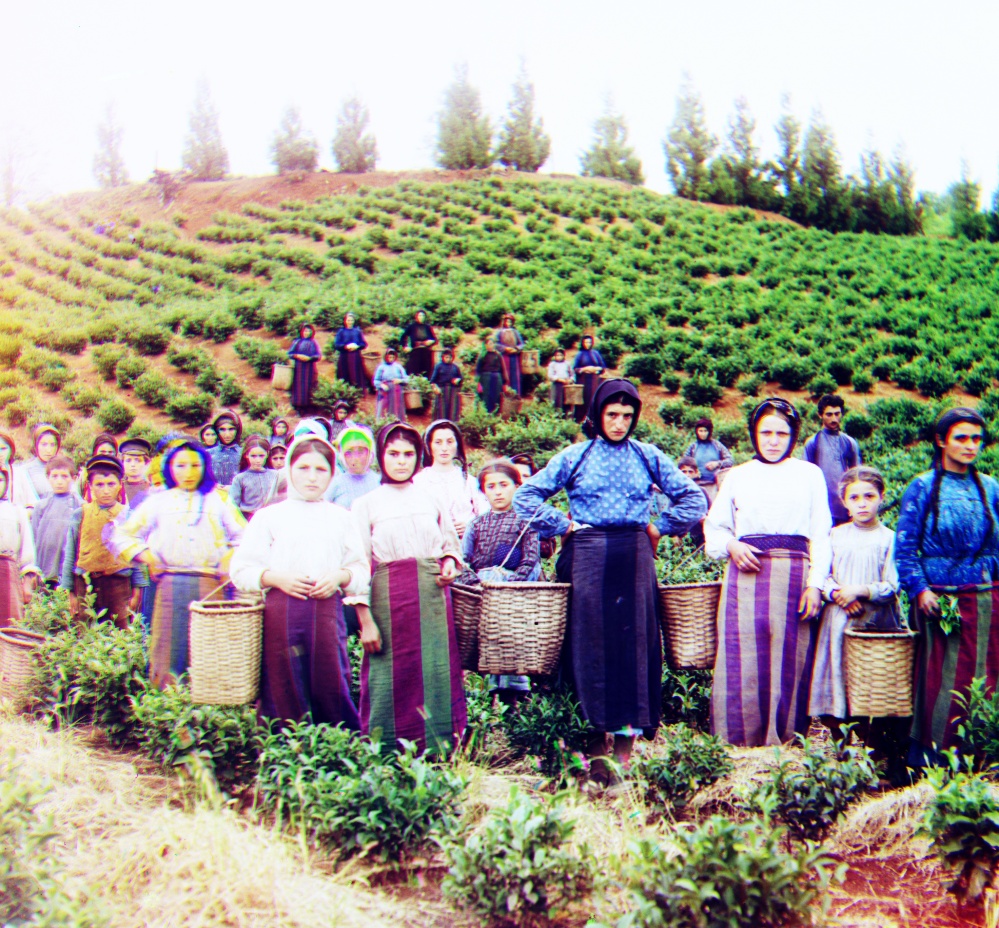
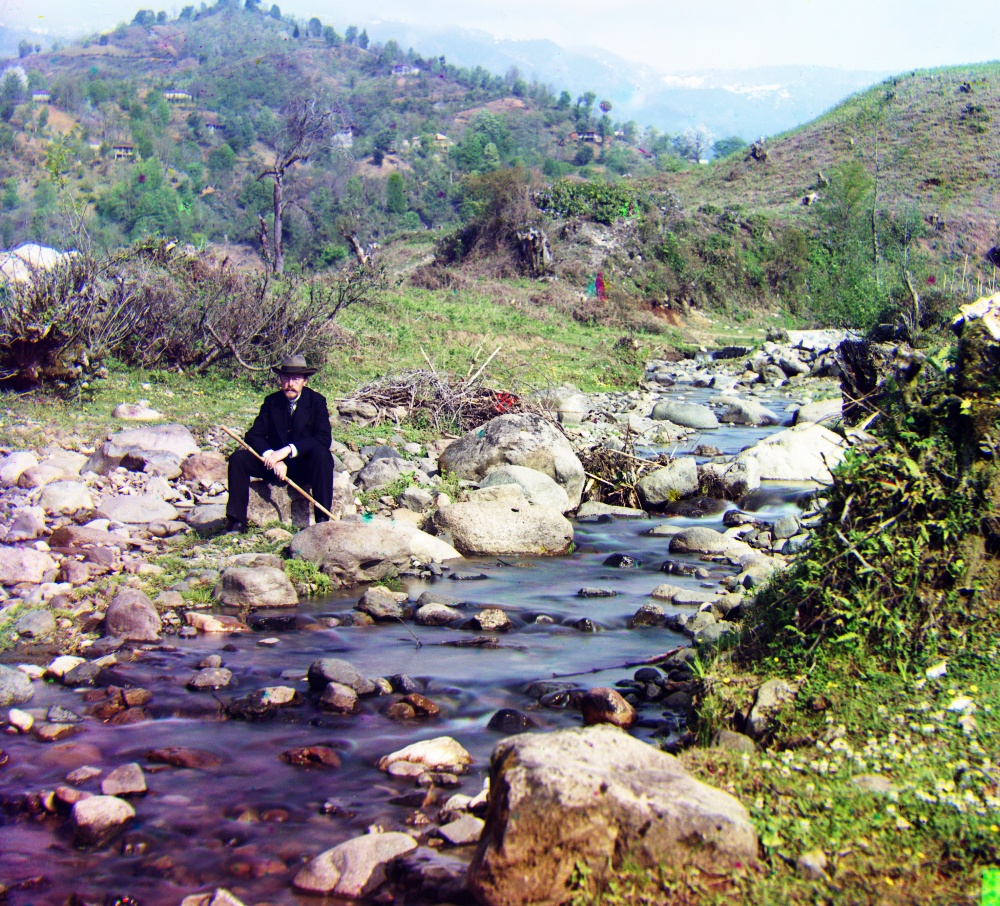
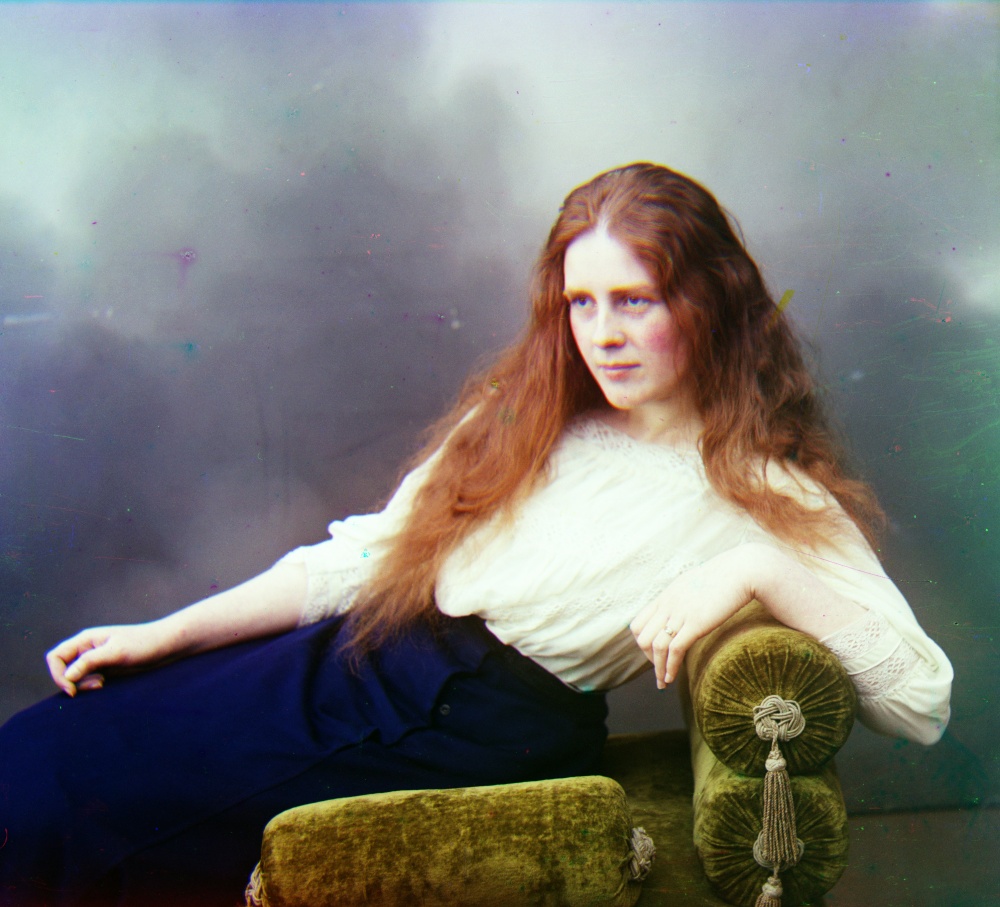

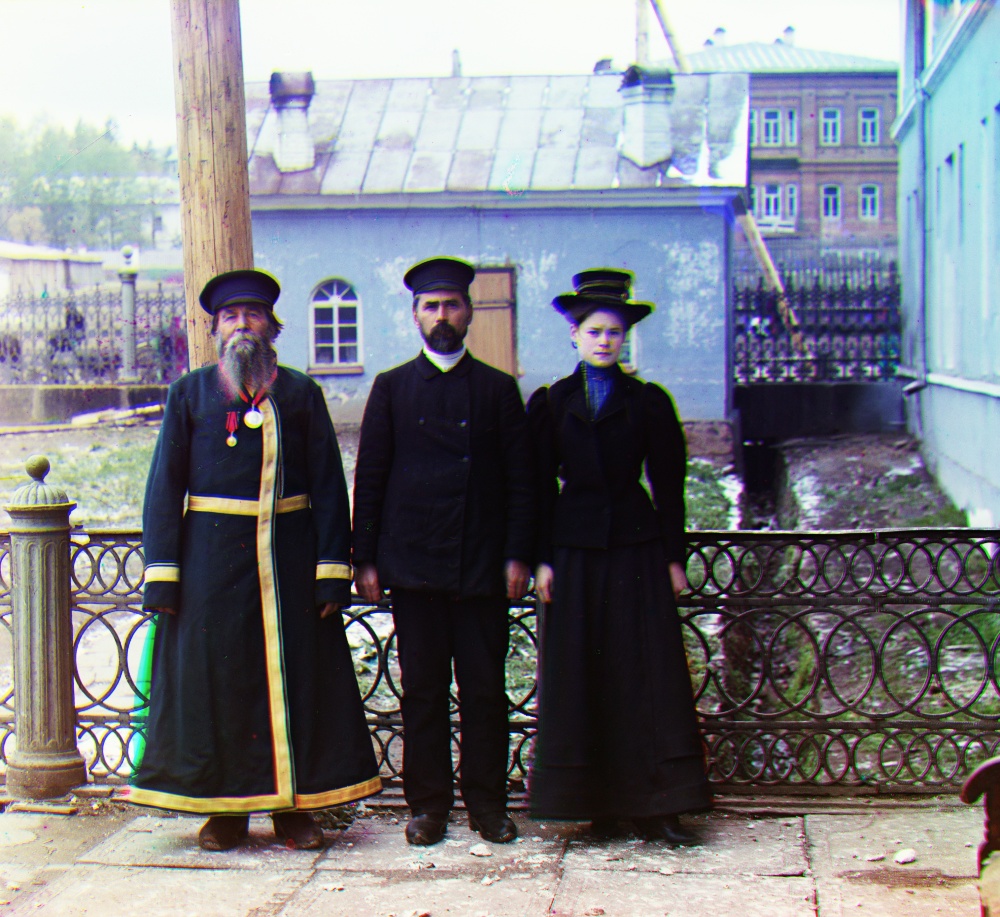
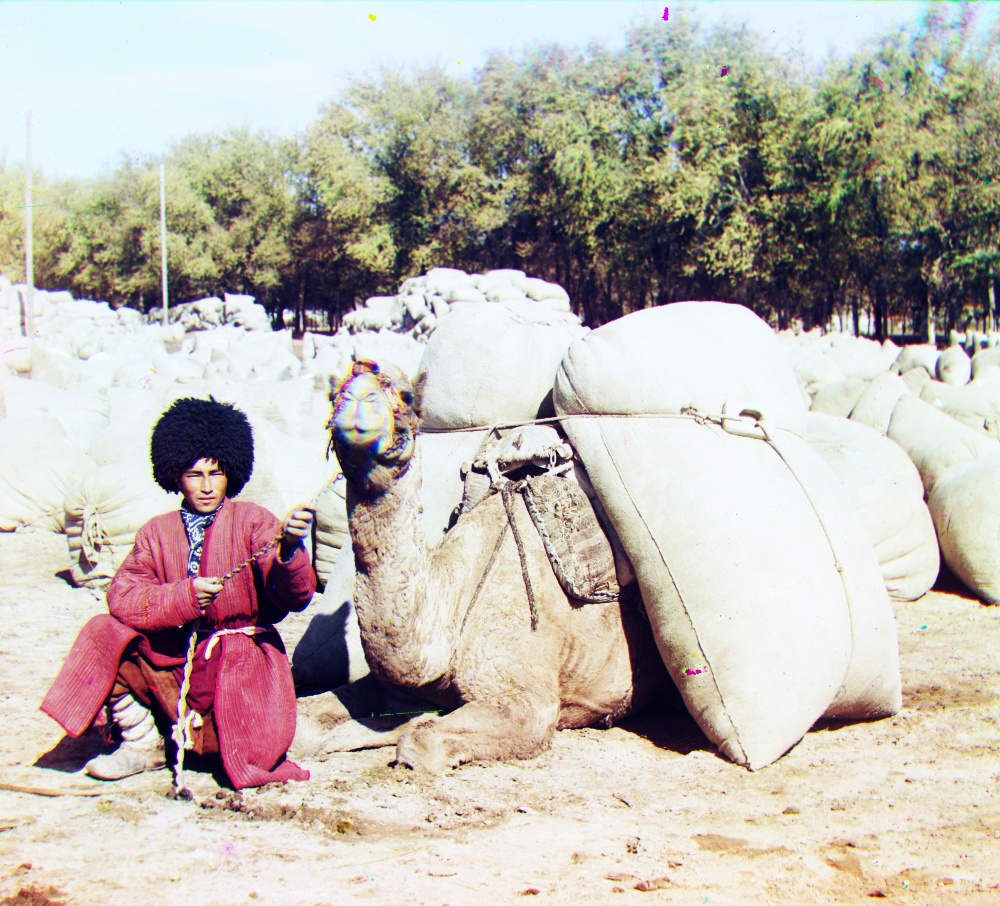
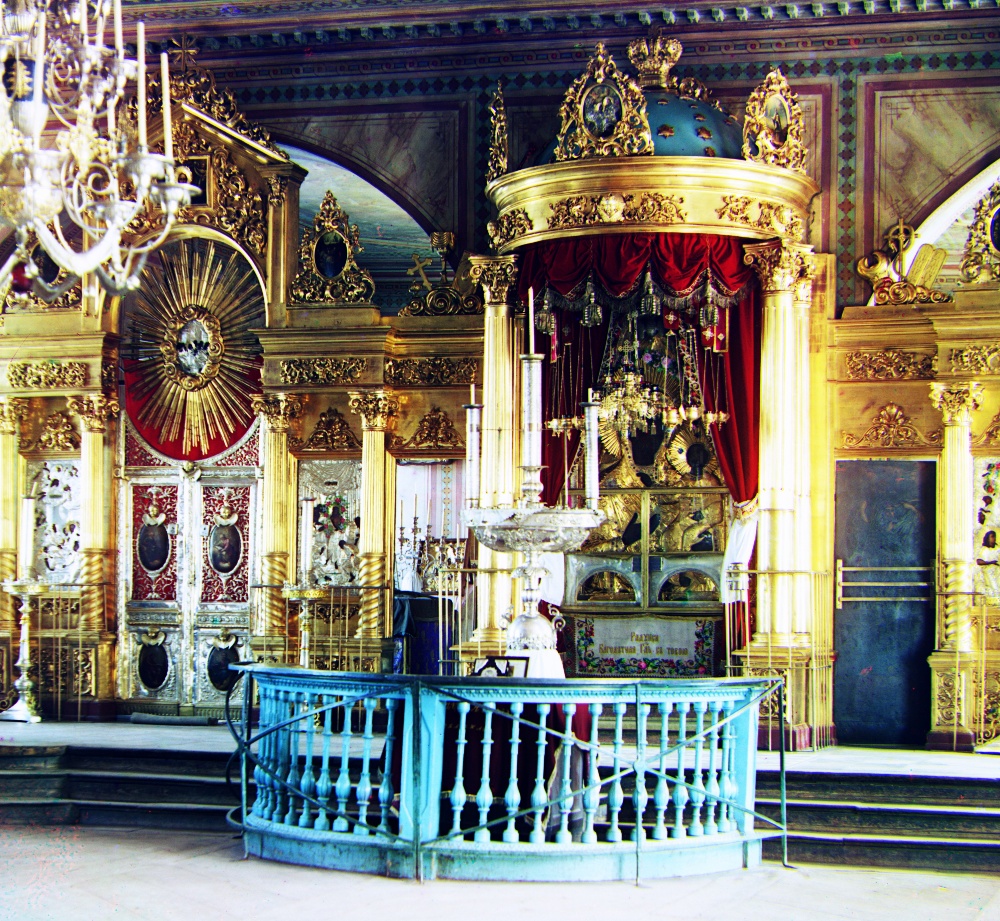
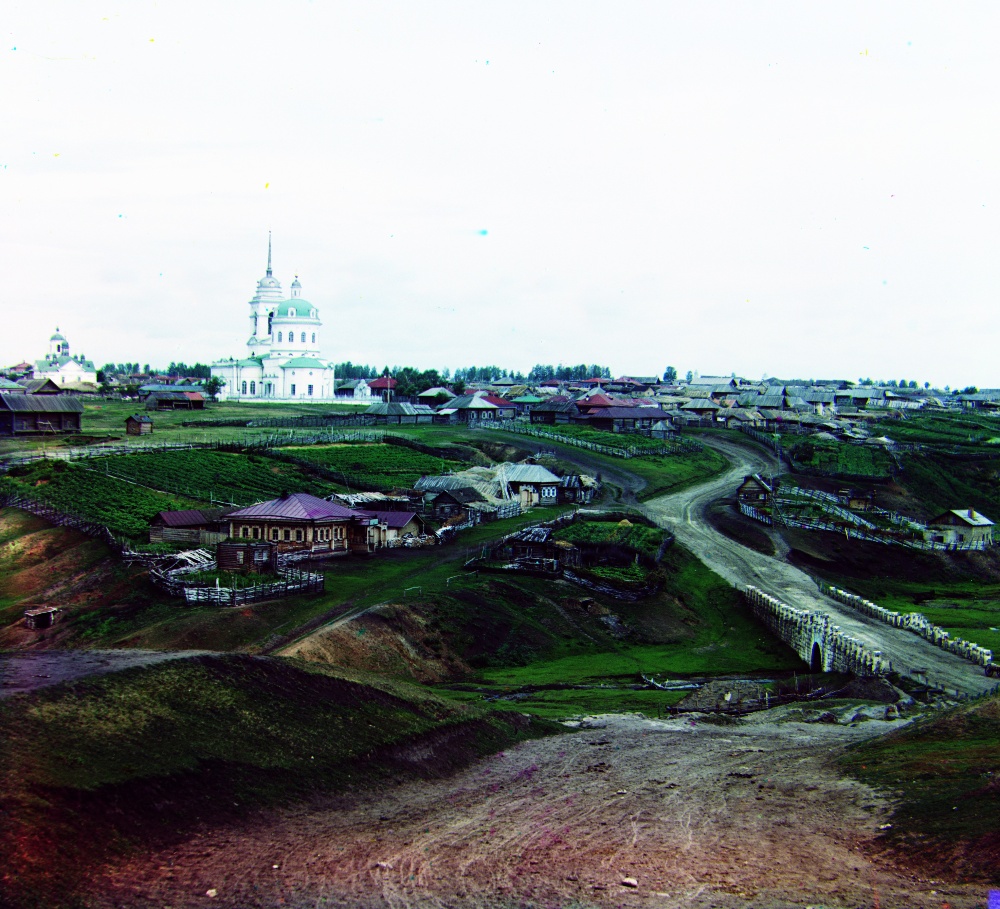

Name | Green channel shift (dy, dx) | Red channel shift (dy, dx) |
---|---|---|
Cathedral | (5, 2) | (12, 3) |
Emir | (49, 23) | (107, 40) |
Harvesters | (60, 17) | (124, 10) |
Icon | (40, 16) | (88, 22) |
Lady | (56, 10) | (120, 13) |
Self-portrait | (77, 29) | (175, 37) |
Three Generations | (56, 12) | (115, 12) |
Train | (43, 1) | (85, 29) |
Turkmen | (57, 22) | (117, 29) |
Village | (65, 11) | (137, 21) |
Extra Images
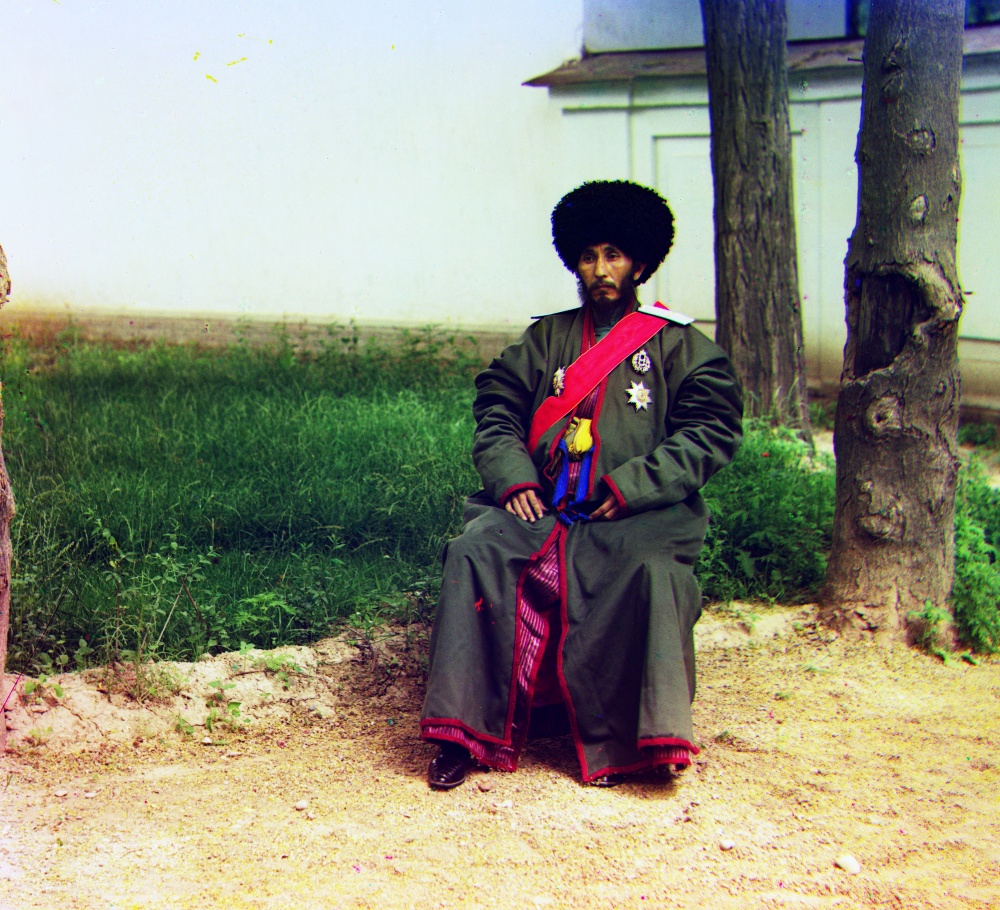

Summary: Extra Features
- PyTorch Implementation
- Edge Alignment
- Auto Contrasting
- Auto Color Mapping